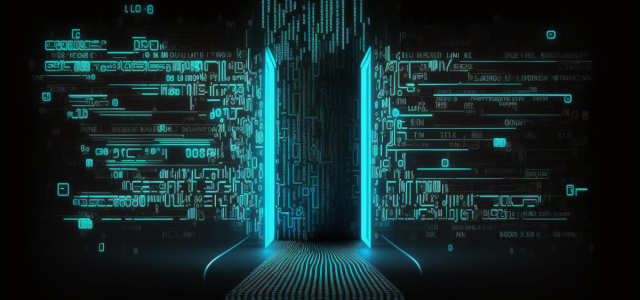

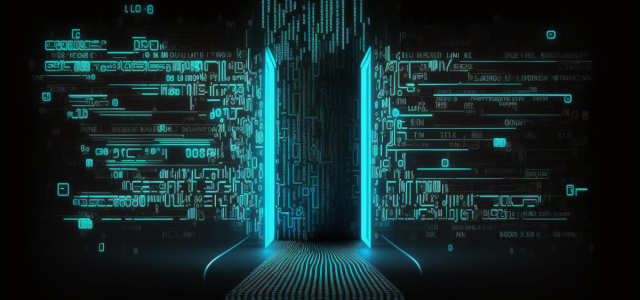
Join top executives in San Francisco on July 11-12, to hear how leaders are integrating and optimizing AI investments for success. Learn More
Leave any preconceptions you may have about AI at the door. If you can get past the futuristic image that the media constructs about AI, you can find real business value: machine learning (ML) models that solve real-world business problems.
From cybersecurity, governance and compliance, and accounting to navigating a recession and managing data, talent, and workloads, AI is here to stay. Its main goals are automation, agility and speed. The limitations of human performance and the impact of human error are unquestionably top AI innovation drivers.
Verizon’s 2022 data breach investigations stated that 82% of 23,000 global cyber incidents analyzed were caused by human errors. But while data analysts and even modern software management solutions are quick to blame humans for mistakes and incidents, there are more complexities at stake.
What exactly are human errors, and why do they occur? The answer to this question is vital. Understanding the root causes of human errors is how AI and risk management frameworks work to minimize disruptions.
Event
Transform 2023
Join us in San Francisco on July 11-12, where top executives will share how they have integrated and optimized AI investments for success and avoided common pitfalls.
How AI can help press the right button
A slip, a lapse, a mix-up. Who has not pressed the wrong button when doing a repetitive task, even if they are highly skilled? Unintentional errors are common in a wide range of industries. They occur in environments where procedures and processes are well-established and automated.
Measuring human error’s global economic and social impact on all industries is a virtually impossible task. But we can rapidly visualize the severe risks involved when, for example, we meditate on the consequences of human error in sectors like healthcare, where lives are on the line. Even Chernobyl — one of the most dangerous nuclear incidents in modern history — began with a human error, followed by a flawed risk management plan.
>>Don’t miss our special issue: The quest for Nirvana: Applying AI at scale.<<
Unintentional human errors can slow performance, disrupt normal production operations and even lead to injuries and death. In response, smart industrial AI-driven platforms are used to detect irregularities in production and distribution systems and flag them before they occur.
How do these platforms work? In the fourth industrial revolution, automation is powered by a network of industrial IoT devices that constantly relay data to an edge gateway, which in turn uploads it to the cloud. In the cloud, AI systems analyze the data for rapid visualization, risk prevention and predictive analysis.
These AI systems can “learn” and improve performance by removing gaps while “fixing” the root causes that lead to human errors.
On the other hand, mistakes also occur when workers are subject to stressful conditions and experience burnout. “Everyone can make errors no matter how well trained and motivated they are,” says the Health and Safety Executive (HSE), Britain’s national regulator for workplace health and safety.
How ML models are built to mitigate impacts on workforces
Unintentional human errors are not only impacting companies. A recent report by BMC Health Services Research found that medication errors were impacting patients directly, and significantly affecting the healthcare staff involved.
The BMC study adds that these errors even drove top health professionals to question their competence. Guilt, fear, self-blame, self-victimization, moral distress and the stigma associated with human errors haunt healthcare workers.
But how are AI error-minimization applications built? When data scientists are called on to build ML models that can predict errors, disruptions and accidents, they will dive into the incidents in a company’s history and search for patterns. For example, they might look into data that reveals a factory line is experiencing power surges, equipment that is not well maintained or workers who are putting in too many hours.
ML models can use this critical data and, through algorithms, predict human errors before they happen. The most advanced models can also come up with innovative solutions.
Understanding decision-making errors and the real cost
Another category of mistakes is those made with good intentions. When humans are confronted with something new, they tend to fall back on their known skills and training. Making assumptions in new environments often leads to human error, even when the person believes he or she is doing the right thing.
For example, while providing endless benefits for companies, the global cloud migration forced IT teams to adapt or die. The digital transformation race led to numerous cloud misconfigurations.
The IBM “Cost of a data breach 2022” report, titled “A million-dollar race to detect and respond,” revealed that after phishing and credential theft (also human error-related), cloud misconfigurations accounted for 15% of all breaches. The average cost for cloud misconfiguration breaches was an astounding $4.14 million per incident. Knowledge gaps concerning the deployment of third-party software and its vulnerabilities totaled 13% of all breaches.
Oversights in cloud credentials, cloud misconfigurations, lack of compliance and governance integration, and the inability to implement the most advanced security practices have had severe consequences for companies. These errors happen not because IT workers acted with malice but because they lacked the necessary skills.
How top cloud vendors pave the way
How can AI minimize human error in the cloud? All top cloud vendors, from Google Cloud Platform (GCP) to Amazon Web Services (AWS) and Microsoft Azure Cloud, have built-in AI features that can automatically consolidate and integrate compliance; check for misconfigurations and network and credential errors; and identify common data mistakes.
These AI solutions can also manage visibility and analytics to enable quicker identification and investigation to resolve issues faster. Cloud AI data features check for format errors, duplicated or inaccurate data, inconsistency and other singularities. Furthermore, they can scan massive Big Data in seconds, which would manually take hours or even days.
Bias and frequency illusion: Finance turns to machine learning
Have you ever noticed that when you are thinking about a specific car model you are interested in buying, you see it everywhere? This is known as the frequency illusion or the Baader–Meinhof phenomenon, LightHouse explains.
Scientists have proven that the human brain tricks us through a mechanism called confirmation bias — the tendency to only seek information that supports our position or idea. Other forms of bias are linked to cultural perceptions, while still others are even more dangerous and cross ethical and legal lines, meeting the definition of discrimination.
Aaron Klein of the Brookings Economic Studies program explains that AI is an opportunity to reduce bias errors in finance and transform the way the industry allocates credit and risk. AI has the ability to create an alternative to the traditional credit reporting and scoring system that helps perpetuate existing bias, Klein says. However, ML models are not designed, built and trained in a vacuum. Neglecting to include ethics, fairness and transparency in ML models can also result in biased AI applications.
Removing bias from the finance industry — “where poor-quality credit (high-interest rates, fees [and] abusive debt traps) and concerns over the usage of too many sources of data … can hide as proxies for illegal discrimination,” as Klein explains — can be done by training AI algorithms and feeding them the right set of data.
Managing human error: Risk assessment frameworks and AI
From deviations in specific rules, regulations and processes to non-compliances, circumventions, shortcuts and workarounds: Human errors and violations will continue to occur.
The good news is that errors are predictable. While AI and ML models can help minimize them, companies should include workers in the design of tasks and procedures and build holistic risk assessment frameworks that better manage human error.
Treating operators as superhuman, overworking talent, making wild assumptions about your personnel, assuming your people will always follow procedures no matter what, lack of proper work conditions and other failures, are the roots and origins of human errors. The responsibility of minimizing incidents is not to be placed on a modern AI application. It should rest on the shoulders of top decision-makers and never on ground-floor or front-line workers.
Risk management and AI are helping doctors better diagnose and treat patients; reducing accidents and disruptions in intelligent factories and industries; transforming supply chains and finance; and boosting cybersecurity. AI can go beyond each individual mistake. It can coldly and unemotionally identify the root cause, predict with accuracy, and propose solutions. However, it takes more than just AI. A profound shift in the way we perceive human errors is the first step on the journey.
Taylor Hersom is founder and CEO of Eden Data.
DataDecisionMakers
Welcome to the VentureBeat community!
DataDecisionMakers is where experts, including the technical people doing data work, can share data-related insights and innovation.
If you want to read about cutting-edge ideas and up-to-date information, best practices, and the future of data and data tech, join us at DataDecisionMakers.
You might even consider contributing an article of your own!